Turning Over MTA Data
Finding the best subway station to loiter in
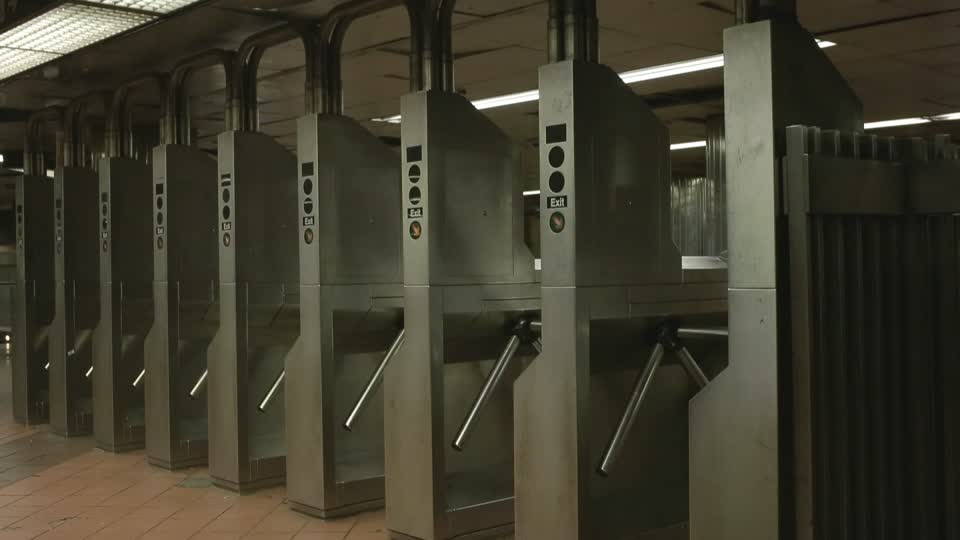
For my first data science project at Metis, I worked with a group tasked finding the best MTA station for the purpose of placing a kiosk and promoting a Women in Technology gala.
Goal
The focus of the project was to use MTA turnstile data in order to find the optimal station. To this end we decided that the goal of the kiosk should be to find the highest quantity of high quality attendees.
Approach
The quantity was defined simply as the foot traffic in a station. The quality was defined as the median donation value of that area. This donation value data came from a 2012 study from a different organization. Because the gala is scheduled for the Summer, we chose data from April and May to promote find the best time to promote the event.
Our methodology was to first find the top 5 foot traffic stations, then cross them with the median donation value for their respective areas.
Results
The top 9 Stations were as follows. The 86th St, 125 St, and 96 St stations were dropped due to the way the data was aggregated. Those stations have multiple lines with the same names but different locations. As a result the stations would aggregate foot traffic counts across multiple locations.
Next we crudely took a product of the media contribution and the foot traffic to get some idea of the overall quality of a station.
We ordered them by our “quality” metric and the Wall St station turned out to be our best bet. So we broke Wall St’s foot traffic down by the week to find a best day to send the street team.
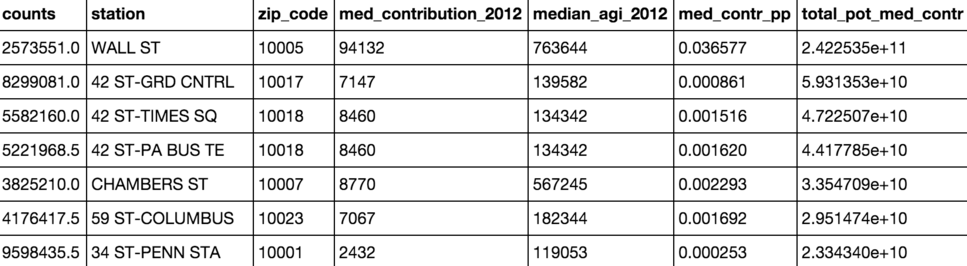
It appears Wednesdays or Thursdays are our best bet. It turns out that other stations in the top 7 appear to follow a similar traffic trend.
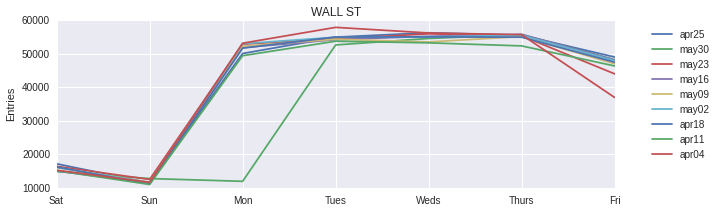
Some limitations of the analysis include the fact that median contributions for a location and MTA may only be loosely connected. Furthermore things catching a lot of people in a hurry were not considered in the analysis.
Data Stuff
From my novice data cleaning perspective it was a significant challenge to correct the often screwed up turnstile data. It was necessary to do things such as interpolating values when turnstiles reset, or deleting values when they bizarrely went backwards. Automating all that was a nice learning experience.